How to Implement an Effective Clinical Data Management System: A Comprehensive User Guide
Clinical data management (CDM) is vital for maintaining the quality and integrity of data collected during clinical trials. In a world where data drives decisions, understanding CDM can make a significant difference in your research's success. Whether you are a researcher, a clinical trial manager, or a data analyst, grasping the fundamentals of CDM will empower you to navigate the complexities of clinical research effectively.
Understanding Clinical Data Management
Clinical data management involves the collection, cleaning, and management of data obtained from clinical trials. Accuracy is essential because the data influences regulatory compliance and patient safety. Establishing well-defined protocols, employing suitable technologies, and adhering to regulations are all key to managing the clinical data lifecycle successfully.
In today's fast-paced environment, organizations must invest in robust methodologies. Without proper CDM practices, the risk of data inaccuracies can rise, potentially leading to significant setbacks in clinical trials.
Key Components of Clinical Data Management
1. Data Collection
Data collection is the first critical step in clinical data management. Selecting the right methods can significantly impact the quality of your data. Common data collection methods include:
Electronic Data Capture (EDC): Streamlined and efficient, it allows for real-time data collection.
Case Report Forms (CRFs): These standardized forms gather essential data from study participants.
Patient Surveys: A vital source of subjective data, aiding in better understanding patient experiences.
Wearable Technology Data: Incorporating data from devices can enhance study engagement and provide continuous monitoring.
Gathering high-quality data is essential for meeting regulatory standards, enhancing the reliability of your results.
2. Data Validation
Once collected, data validation is crucial for ensuring its accuracy and completeness. Effective validation techniques might include:
Range Checks: Ensuring that values fall within expected limits; for example, a patient's age must be a positive integer.
Consistency Checks: Cross-referencing related data fields to confirm that information aligns.
Duplicate Checks: Implementing algorithms to detect and address repeated data entries is vital for maintaining data integrity.
By applying these techniques, you can significantly reduce the chances of errors impacting the study results. Studies indicate that effective data validation can improve data reliability by up to 30%.
3. Data Cleaning
Data cleaning is necessary to identify and correct errors or inconsistencies. Key cleaning processes include:
Removing Duplicates: Streamlining your dataset by eliminating repeated entries.
Filling Missing Values: Using statistical methods to estimate and fill gaps where data may be incomplete.
Correcting Erroneous Entries: Reviewing data for inaccuracies through automated checks and peer review.
Employing software tools designed for data cleaning can increase productivity by up to 40%, allowing your team to focus on more challenging issues.
4. Data Storage
After validating and cleaning your data, secure storage is essential. Considerations for effective data storage include:
Secure Servers and Databases: Using encrypted databases protects sensitive information from unauthorized access.
Regular Data Backups: Scheduling backups minimizes the risk of data loss, with many organizations conducting daily backups.
Proper Documentation: Creating detailed protocols ensures that everyone can access the information securely.
An efficient storage solution not only preserves data integrity but also simplifies access for future analysis and reporting.
5. Data Analysis
Data analysis transforms raw data into meaningful insights. During this stage, organizations should:
Select Appropriate Statistical Methods: Tailoring your approach to suit the data type can enhance clarity. For example, using regression analysis for continuous data.
Utilize Software Tools: Tools such as SAS, R, or Python facilitate data manipulation and statistical analysis.
Involve Statisticians: Engaging professionals during the analysis phase ensures accurate interpretations of the data. Their expertise can lead to clearer insights and better decision-making.
Proper data analysis is critical, as around 70% of research findings can depend on the methods used during this phase.
6. Reporting
Effective reporting communicates research findings clearly and transparently. This step can include:
Clinical Study Reports (CSRs): Comprehensive documents summarizing clinical trial methods and results.
Manuscripts for Publication: Ensuring that your work reaches the scientific community to foster further research.
Presentations at Conferences: Sharing insights with peers encourages collaboration and knowledge exchange.
Clear reporting ensures that findings are understood and disseminated appropriately, influencing future clinical practices.
Selecting the Right Clinical Data Management Software
Features to Look For
Choosing suitable clinical data management software can be a complex decision. Key features to consider include:
User-Friendly Interface: An intuitive design encourages team members to use the system effectively.
Electronic Data Capture (EDC): Allows seamless integration with participant data collection processes.
Automated Validation Checks: Built-in rules help prevent human errors, enhancing data quality.
Regulatory Compliance: Ensure the software adheres to FDA, GCP, and ICH guidelines.
Customizable Reporting Capabilities: Flexibility in report generation can streamline the presentation of findings.
Popular CDM Software
Medidata Solutions: Known for its comprehensive suite tailored for clinical trials.
Oracle's Siebel CTMS: Offers robust tools for clinical trial management.
OpenClinica: A valorous choice for open-source data management.
Veeva Vault CDMS: Renowned for its focus on compliance and usability.
Conducting thorough research and possibly pilot testing different systems helps determine which software best aligns with your clinical trial requirements.
Developing Standard Operating Procedures (SOPs)
Establishing clear Standard Operating Procedures (SOPs) is vital for ensuring consistency and compliance in CDM. SOPs should cover:
Data Collection Protocols: Outlining specific procedures for capturing data accurately.
Data Validation Techniques: Providing guidelines for maintaining high data quality.
Data Cleaning Procedures: Ensuring standardized practices for correcting data.
Data Security Measures: Detailing how to protect sensitive information.
Reporting Methodologies: Ensuring consistent communication of findings.
Importance of Training
Training team members on established SOPs is crucial for success. Regular training sessions ensure all staff understand their roles, adhered to procedures, and maintain high standards throughout the data management process.
Compliance and Regulatory Considerations
Compliance with regulations significantly impacts clinical data management. Organizations must ensure alignment with:
Good Clinical Practice (GCP): Ensures that clinical trials are conducted ethically.
International Conference on Harmonisation (ICH) Guidelines: Provides guidelines for harmonized regulatory requirements.
FDA Regulations: Adheres to necessary safety standards for human subjects.
Maintaining compliance not only enhances the credibility of your study but protects the rights and welfare of all participants involved.
Auditing and Quality Control
Regular audits and quality control measures throughout the clinical data management process help identify potential issues early. Doing so ensures data integrity is upheld, significantly reducing the chance of regulatory penalties.
Leveraging Technology in Clinical Data Management
Integrating emerging technologies can enhance clinical data management efficiency. Consider these innovative solutions:
1. Artificial Intelligence (AI)
AI can be a game-changer in CDM by providing insights into data anomalies and automating routine tasks, which can reduce labor time by approximately 20% in many cases.
2. Blockchain
Blockchain technology offers a secure and transparent method for recording and sharing clinical data. This enhances trust among stakeholders and improves data integrity, as each transaction is immutable.
3. Cloud Computing
Cloud-based CDM systems provide scalability and adaptability to changing data requirements. They offer quick access to data while ensuring robust security measures are in place.
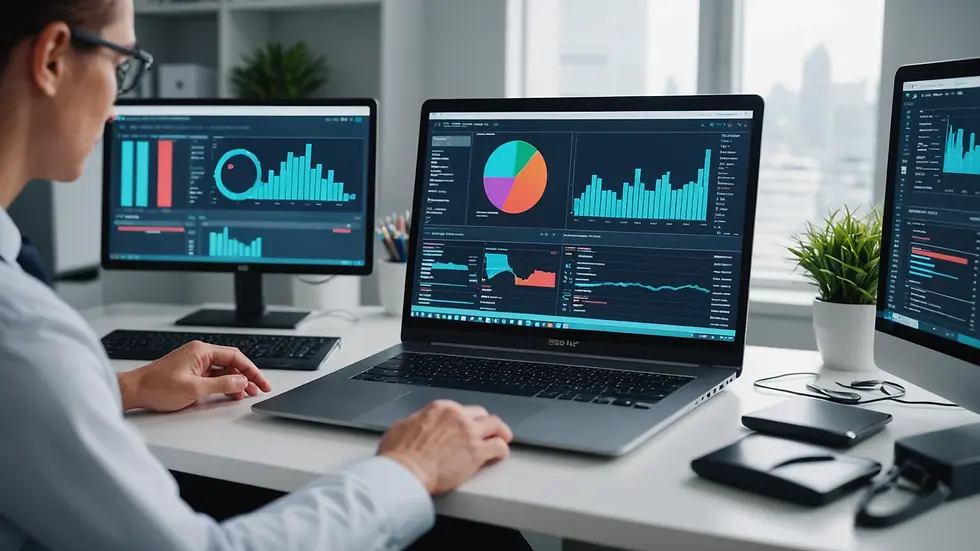
Final Thoughts
Implementing an effective clinical data management system is an ongoing process that requires commitment, training, and flexibility. Focusing on key components such as data collection, validation, cleaning, storage, analysis, and reporting ensures the integrity of clinical trials.
By investing in the right software, establishing robust SOPs, maintaining compliance, and leveraging advanced technologies, organizations can build a strong foundation for successful data management.
As clinical research continues to evolve, staying aware of emerging trends and innovations will enhance your organization’s capabilities, ultimately leading to better outcomes for clinical trials and improved patient care.
Comentarios